Can a Butterfly Cause a Hurricane? Butterfly Effect!
You’re dealing with the concept of the Butterfly Effect, rooted in Chaos Theory by Edward Lorenz. It shows how tiny changes in initial conditions can lead to vastly different outcomes.
Realistically, the probability is exceedingly low due to dominant large-scale factors like sea surface temperatures.
However, this metaphor underscores the sensitivity and unpredictability in weather forecasting. To grasp the full complexity of these ideas, there’s a lot more to explore in the domain of chaos and meteorology.
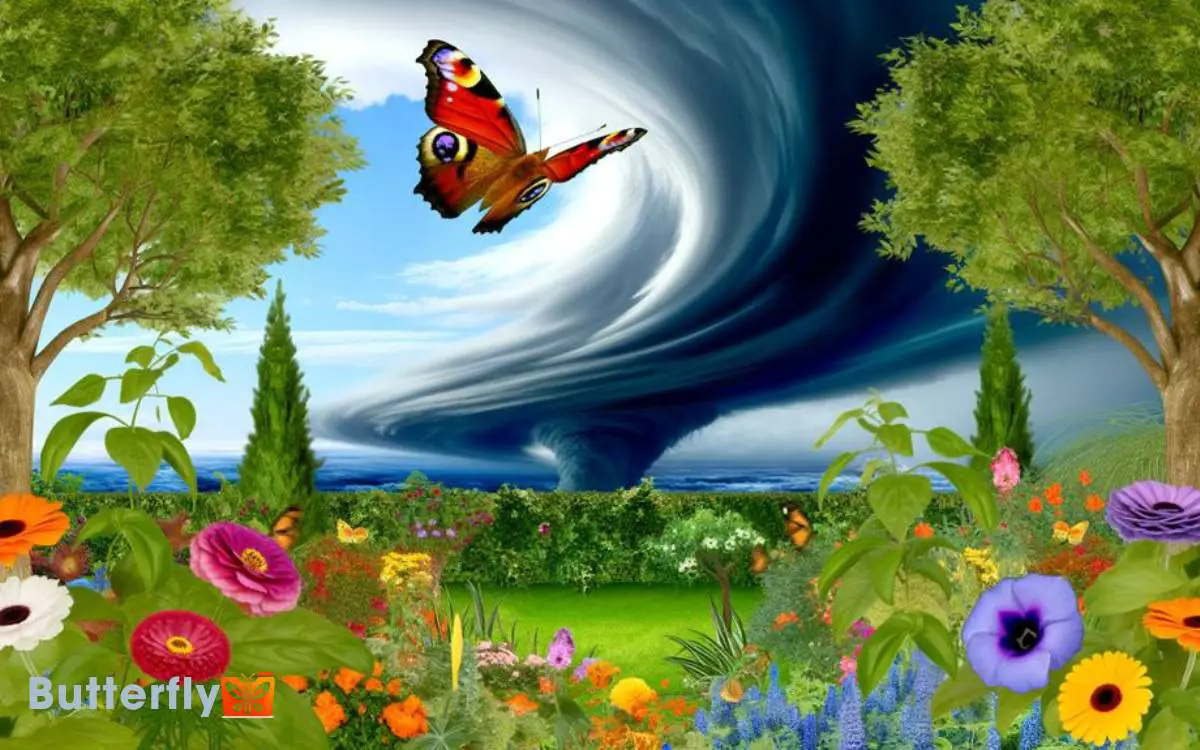
Key Takeaways
The Origin of the Butterfly Effect
The concept of the Butterfly Effect originated from meteorologist Edward Lorenz‘s work in the 1960s, where he discovered that small changes in initial conditions could lead to vastly different outcomes in weather models.
You’ll find that Lorenz noticed even minor fluctuations in data input, such as rounding errors, could drastically alter the predicted weather patterns. This phenomenon underscores the sensitivity of complex systems to initial conditions.
Lorenz’s 1963 paper, ‘Deterministic Nonperiodic Flow,’ provided empirical evidence and mathematical proof for this sensitivity, using differential equations and data simulations.
By demonstrating that tiny variations could amplify over time, Lorenz highlighted the inherent unpredictability in forecasting systems, emphasizing the need for precise initial data in predictive models.
Understanding Chaos Theory
At its core, Chaos Theory examines how small changes in a system’s initial conditions can lead to vastly divergent outcomes, rendering long-term predictions inherently unreliable.
You can think of it as the sensitivity of complex systems to initial conditions. In mathematical terms, even minuscule changes can exponentially amplify over time, creating unpredictability.
Consider the following data:
Initial Condition | Time (T=1) | Time (T=10) |
---|---|---|
0.0001 | 0.002 | 0.8 |
0.0002 | 0.003 | 1.2 |
0.0003 | 0.004 | 2.5 |
This table illustrates how tiny initial differences, such as 0.0001 vs. 0.0002, can lead to vastly different states over time. Chaos Theory highlights the challenges in predicting the behavior of dynamic systems.
Lorenz’s Weather Model
You should analyze Lorenz’s Weather Model to understand chaotic weather patterns and their sensitivity to initial conditions.
This model uses differential equations to simulate atmospheric dynamics and reveals how minor changes can lead to vastly different outcomes.
Chaotic Weather Patterns
In examining Lorenz’s weather model, you’ll encounter the foundational principles of chaotic weather patterns, where minute changes in initial conditions can lead to vastly different outcomes.
The model, based on nonlinear differential equations, showcases how small perturbations can evolve unpredictably.
By analyzing datasets of temperature, pressure, and wind speed, you’ll see how these variables interact in complex, non-linear ways.
Lorenz’s attractors illustrate the system’s sensitivity, revealing patterns that never exactly repeat yet remain within a bounded region. Such behavior underscores the intrinsic unpredictability of long-term weather forecasting.
Through computational simulations, you’ll observe how initial state variations result in divergent weather scenarios, emphasizing the model’s chaotic nature and its implications for meteorological predictions.
Sensitivity to Initial Conditions
Lorenz’s weather model starkly demonstrates how even the slightest change in initial conditions can exponentially amplify, leading to vastly different weather outcomes.
You see, Lorenz discovered this sensitivity when he found that minute variations in initial data could produce drastically divergent results.
This phenomenon is essential for understanding chaotic systems like weather. Here’s why:
- Nonlinearity: The model’s equations are nonlinear, meaning small changes can lead to unpredictable outcomes.
- Exponential Growth: Tiny initial differences grow exponentially, making long-term forecasting nearly impossible.
- Deterministic Chaos: Even though the system is deterministic, it behaves unpredictably over time.
Understanding these points helps you grasp why weather prediction is so challenging and why even the flutter of a butterfly’s wings could theoretically influence a hurricane’s formation.
Mathematical Modeling Insights
Building on the concept of sensitivity to initial conditions, Lorenz’s weather model offers profound insights into the chaotic behavior of atmospheric systems through its set of nonlinear differential equations.
You’ll find that these equations describe how variables like temperature, pressure, and wind speed evolve over time. By solving them numerically, you can observe that even minuscule changes in initial conditions lead to vastly different outcomes.
This phenomenon, known as deterministic chaos, underscores why long-term weather prediction is so challenging.
Lorenz’s model, specifically the Lorenz attractor, visually demonstrates how these variables don’t converge to a steady state but rather form a complex, fractal-like pattern.
This insight emphasizes the inherent unpredictability and complexity of weather systems.
Small Changes, Big Impacts
The concept of ‘sensitive dependence on initial conditions’ illustrates how minute alterations in a system’s starting state can exponentially influence its long-term behavior. This principle, fundamental to chaos theory, underscores the significance of even the smallest changes.
For instance, consider:
- Weather Systems: A tiny change in atmospheric pressure can drastically alter weather outcomes.
- Population Dynamics: Slight variations in birth rates can lead to significant shifts in population demographics over time.
- Financial Markets: Minor fluctuations in market conditions can trigger substantial economic impacts.
You can see that these small changes can have cascading effects, making predictions exceedingly complex. Understanding these dynamics is important for developing more accurate models and enhancing our ability to manage and respond to various systems effectively.
Meteorology and Predictability
When it comes to meteorology, sensitive dependence on initial conditions makes weather forecasting a formidable challenge, as even a minuscule variation in data can lead to vastly different outcomes.
You must consider the chaotic nature of atmospheric dynamics, where slight changes in temperature, pressure, or humidity can cascade into unpredictable effects. This phenomenon, often described as the Butterfly Effect, underscores the complexity of predictive models.
Emotionally Charged Data:
Factor | Impact on Forecasting |
---|---|
Temperature Variations | Can alter storm paths |
Pressure Changes | May intensify hurricanes |
Humidity Fluctuations | Affects precipitation rates |
Accurate data collection and sophisticated algorithms are essential, but achieving perfect predictability remains elusive. Understanding these dynamics helps appreciate the intricacies involved in forecasting efforts.
Real-world Examples
You can see chaotic weather patterns manifest in numerous historical meteorological events, demonstrating the butterfly effect’s principles.
For instance, the 1972 European floods were influenced by minor atmospheric changes, highlighting unpredictable outcomes.
Analyze data from the 2004 Atlantic hurricane season to further understand how small initial conditions can escalate into significant weather phenomena.
Chaotic Weather Patterns
In 1961, meteorologist Edward Lorenz discovered that small changes in initial atmospheric conditions could lead to vastly different weather outcomes, exemplifying the chaotic nature of weather systems.
This phenomenon, known as the Butterfly Effect, underscores the sensitivity of weather models to initial conditions.
Here are three real-world examples showcasing chaotic weather patterns:
- European Heatwave (2003): Minor atmospheric variations contributed to one of the deadliest heatwaves, causing approximately 70,000 fatalities.
- Superstorm Sandy (2012): Slight shifts in ocean temperatures and wind patterns escalated this storm’s intensity, affecting millions along the U.S. East Coast.
- Indian Monsoon Variability: Annual monsoon patterns show significant interannual variability due to small changes in sea surface temperatures and atmospheric pressure.
These cases highlight the inherent unpredictability and complexity of weather systems.
Historical Meteorological Events
Examining historical meteorological events, such as the Galveston Hurricane of 1900 and the Dust Bowl of the 1930s, reveals how small atmospheric variations can escalate into catastrophic weather phenomena.
The Galveston Hurricane, with winds exceeding 145 mph, started as a tropical disturbance. Minor shifts in pressure and temperature intensified it into a Category 4 hurricane, resulting in over 6,000 fatalities.
The Dust Bowl, marked by severe dust storms, was triggered by drought and poor agricultural practices. Small-scale atmospheric changes, like reduced rainfall, led to large-scale soil erosion.
These events illustrate how seemingly minor atmospheric anomalies can amplify into disastrous outcomes, highlighting the intricate sensitivity of weather systems to initial conditions.
Limitations of Weather Models
Weather models often struggle with accurately predicting long-term events due to the inherent complexity and chaotic nature of atmospheric systems.
You need to understand that even small errors in initial conditions can lead to drastically different outcomes. This is known as the butterfly effect.
Here are three key limitations:
- Resolution: Higher resolution models offer more detail but require immense computational power, often limiting their usage.
- Incomplete Data: Data gaps and inaccuracies from remote or under-sampled areas can skew model outputs.
- Parameterization: Simplifying complex processes (like cloud formation) into manageable equations can introduce significant errors.
These limitations make long-term forecasts challenging, emphasizing the need for continuous data refinement and advanced computational methods. Understanding these constraints helps you appreciate the intricacies of meteorological predictions.
Human Contributions to Chaos
How do human activities amplify the inherent chaos in atmospheric systems, further complicating our ability to predict weather patterns accurately?
By increasing greenhouse gas concentrations, you’re altering the Earth’s radiative balance. This drives more extreme weather events, like heatwaves and heavy rainfall, which destabilize established patterns.
Urbanization introduces heat islands, modifying local atmospheric conditions. Deforestation reduces evapotranspiration, affecting humidity levels and wind patterns.
Industrial emissions and aerosols scatter solar radiation, influencing cloud formation and precipitation. These anthropogenic factors inject variability into climate models, making it harder to isolate natural fluctuations.
Your actions contribute to a complex web of interactions, where even minor changes can cascade, magnifying chaos and challenging our predictive capabilities. Understanding these dynamics is crucial for accurate weather forecasting.
Computer Simulations and Predictions
You’ll find that computer simulations leverage complex algorithms to model weather patterns, incorporating principles from chaos theory to account for initial condition sensitivity.
Despite these advanced techniques, predictive accuracy remains a significant challenge due to the inherently unpredictable nature of chaotic systems.
Consequently, small errors in data input can lead to vastly different outcomes, highlighting the limitations of current predictive models.
Modeling Weather Patterns
Computer simulations are indispensable for predicting weather patterns. Meteorologists analyze vast datasets and model complex atmospheric dynamics with remarkable accuracy using sophisticated algorithms and high-performance computing.
These simulations involve processing terabytes of meteorological data. Here’s why they’re essential:
- Data Integration: They synthesize data from satellites, radar, and weather stations, providing a thorough atmospheric snapshot.
- Predictive Models: They employ numerical weather prediction models (NWP) to forecast variables like temperature, wind speed, and humidity.
- Scenario Analysis: They enable the testing of various atmospheric scenarios, helping you understand potential outcomes under different conditions.
These tools transform raw data into actionable insights, enhancing our ability to anticipate weather changes effectively.
Chaos Theory Applications
Understanding the unpredictability in weather forecasting, chaos theory reveals how minor variations in initial conditions can lead to vastly different outcomes in computer simulations.
By applying chaos theory, you can analyze how small perturbations amplify over time, greatly affecting long-term weather predictions.
Advanced algorithms and high-resolution models are essential in capturing these intricate dynamics. For instance, ensemble forecasting uses multiple simulations with slightly different initial states to provide a probabilistic forecast, enhancing your predictive capabilities.
Data from satellites, weather stations, and buoys feed into these models, enabling the evaluation of potential weather scenarios.
You’ll notice that even with sophisticated technology, the inherent sensitivity in initial conditions underscores the complex, non-linear nature of atmospheric systems.
Predictive Accuracy Challenges
Despite advancements in computational power and modeling techniques, achieving high predictive accuracy in weather simulations remains a significant challenge due to the chaotic nature of atmospheric systems.
You’ll find that even minor discrepancies in initial conditions can lead to vastly different outcomes, a phenomenon known as sensitivity to initial conditions. This unpredictability complicates long-term forecasting and underscores the inherent limitations.
To engage with the complexities, consider these factors:
- Model Resolution: Higher resolution models can capture finer details but demand greater computational resources.
- Data Assimilation: Integrating real-time data into models improves accuracy but requires sophisticated algorithms.
- Parameterization: Simplifying complex processes like cloud formation introduces uncertainties that affect predictions.
These challenges highlight why achieving perfect predictive accuracy is elusive, despite technological progress.
Misconceptions About the Butterfly Effect
Many people incorrectly assume that the butterfly effect suggests a butterfly’s wings can directly cause a hurricane, but the concept actually illustrates the sensitivity of complex systems to initial conditions.
When you analyze meteorological models, you’ll find that minor variations in initial data can lead to vastly different outcomes over time.
This phenomenon, known as deterministic chaos, highlights how small perturbations can exponentially amplify, making long-term predictions inherently uncertain.
It’s not about the butterfly causing the hurricane but about understanding how minuscule changes in weather conditions can alter large-scale atmospheric patterns.
Thus, the butterfly effect is a metaphor for the underlying unpredictability in complex systems, emphasizing the need for precise initial measurements and acknowledging the limitations in forecasting.
The Role of Initial Conditions
Recognizing the critical role of initial conditions, meteorologists rely on high-resolution data to improve the accuracy of weather prediction models. You need to understand that even minor inaccuracies in initial conditions can lead to vastly different outcomes.
To capture these nuances, meteorologists utilize:
- Satellite Observations: High-resolution imagery provides real-time data on atmospheric conditions.
- Weather Stations: Ground-based sensors measure temperature, humidity, wind speed, and pressure with high precision.
- Radar Systems: Doppler radar helps in tracking precipitation and storm development.
These tools collectively refine initial conditions, enabling more reliable forecasts.
Can a Butterfly Cause a Hurricane?
Understanding the importance of initial conditions, you might wonder if a butterfly flapping its wings can truly set off a chain of events leading to a hurricane. In chaos theory, this concept is known as the Butterfly Effect.
Small perturbations in a system can amplify over time, but it’s vital to quantify these perturbations.
Meteorological models indicate that while minuscule disturbances like a butterfly’s wings do alter atmospheric conditions, the likelihood of these changes cascading into a hurricane is statistically insignificant.
Data shows that the formation of hurricanes involves multiple large-scale factors such as sea surface temperatures and atmospheric pressure systems. Although theoretically possible, the practical probability remains exceedingly low, underscoring the complexity of weather systems.
Conclusion
You might be surprised to learn that a mere 0.0001% change in atmospheric conditions can drastically alter weather predictions within days. This highlights the profound sensitivity within chaotic systems like our atmosphere.
While a butterfly can’t directly cause a hurricane, its tiny influence on initial conditions can compound over time, leading to significant deviations.
Remember, in meteorology, even the smallest variables matter, making accurate predictions incredibly complex and fascinatingly unpredictable.